Introduction
Fishing is recognized as one of the most hazardous occupations (Jin & Thunberg, 2005), with an estimated 32,000 fatalities occurring annually on fishing vessels worldwide (FAO, 2024). Fishermen are exposed to risks from the hull or fishing gear as well as external dangers such as severe weather and high waves. Despite these dangers, fisheries remain crucial for ensuring future food security. By 2028, global average seafood consumption is estimated to reach 21.3 kg, which is 4.2% higher than the consumption in 2017 (20.4 kg) and is predicted to increase twice as fast as grain or meat consumption (OECD & FAO, 2019).
The International Maritime Organization (IMO) attempted to prevent fishing-related accidents through the 1977 Torremolinos Convention and the 1993 Torremolinos Protocol, but neither was ratified. In 2012, the Cape Town Agreement was introduced to consolidate and revise these earlier agreements, with ongoing efforts to secure ratifications. To enhance maritime safety, the IMO also approved the formal safety assessment (FSA) in 2002 (MSC/Circ.1023/MEPC/Circ.392) and established the current assessment framework in 2018 after several revisions (MSC-MEPC.2/Circ.12/Rev.2)(Fig. 1).
The FSA consists of five steps: hazard identification, risk assessment, development of risk control strategies, cost-benefit analysis, and recommendations for decision-making. The second step, risk assessment, outlines nine analytical techniques for conducting scientific and quantitative risk evaluations (IMO, 2018). Many studies have utilized FSA-recommended analytical methods to examine maritime accidents and safety. For example, previous studies have assessed collision and grounding risks of oil tankers using fault tree analysis (Uğurlu et al., 2015), quantitative risk of maritime accidents in Bangladeshi waters using event tree analysis (Raiyan et al., 2017), risk of passenger ferry fires using failure mode and effect analysis (Jiang et al., 2023), and accident risk of fishing vessels over 7 m using Bayesian network analysis (Uğurlu et al., 2020).
The Bayesian network has been widely used in fields such as reliability, risk analysis, and maintenance and repair. Bayesian belief networks enable modeling of probabilistic data with dependencies between events and are well-suited for risk analysis (Weber et al., 2012). This feature is particularly useful for modeling maritime accidents, as it facilitates quantitative analysis of human and organizational factors (Zhang & Thai, 2016). Research on maritime accidents and safety has predominantly focused on major incidents like collisions, sinkings, and groundings. However, the probability of major accidents involving large vessels is relatively low compared to the potential severity of their outcomes (Elliott et al., 2008). In this study, the risk of industrial accidents faced by fishermen was quantitatively assessed using records of accident insurance claim approvals fishermen in costal and offshore stow net fisheries of Korea between 2018 and 2022.
Materials and Methods
This study utilized data from approved claims under the Compensation Insurance for Fishermen and Vessels in South Korea between 2018–2022 to obtain reliable information on industrial accidents in the coastal and offshore stow net fisheries. This insurance system was implemented in 2004 provides detailed records of accidents, such as the type, size, and age of the vessels; fishermen demographics; and other details of the incidents. Over the 5-year period, 1,193 claims were approved in stow net, providing a robust dataset for quantitative risk assessment.
The insurance data included various factors related to industrial accidents involving fishermen. A chi-square test was conducted to determine whether these factors were statistically associated with the patterns of accidents in coastal and offshore stow net fisheries of South Korea. A chi-square test evaluates whether the difference between observed frequencies (O) and expected frequencies (E) is statistically significant. Qualitative data is used for the chi-square test (Güngör & Bulut, 2008; McHugh, 2013). One of the main advantages of using this test is that it can be applied to both nominal and ordinal data (Burns & Dobson, 1981; Sirkin, 2006). The general hypotheses for the chi-square test are as follows (McHugh, 2013; Sirkin, 2006):
-
- Null hypothesis (H0): The two variables are mutually independent.
-
- Alternative hypothesis (H1 or Ha): The two variables are not mutually independent.
The significance level (p) is was set at 0.05. A p-value > 0.05 indicates that H0 is accepted, whereas a p-value < 0.05 indicates that H1 or Ha is accepted.
The Bayesian network, also known as a belief network or probabilistic directed acyclic graph, is a graphical model that represents the joint probability distribution of a set of variables (Pearl, 1988). Bayesian Networks requires an understanding of conditional probability, which refers to the likelihood of an event occurring given that another event has already occurred. Specifically, the probability of event A occurring given event B is called the “conditional probability of A given B,” expressed as P(A|B), and can change based on event B. Generally, P(A|B) and P(B|A) are not equal. This relationship between the prior and posterior probabilities of two variables can be used to define the relationship between conditional probability and marginal probability (Eq. 1).
Here, P(A) represents the prior probability of event A before event B occurs; P(B) is the prior probability of event B; P(A∩B) is the joint probability of both events occurring; P(A|B) is the posterior probability of event A given event B; and P(B|A) is the likelihood function for event A given event B (Kitson et al., 2023). For example, if the industrial accident rate in coastal and offshore fisheries is denoted as P(I) and the industrial accident rate in stow net fisheries is denoted as P(S), it can be expressed as follows (Eq. 2).
The probability of an industrial accident occurring in stow net fisheries, given that an industrial accident has occurred in coastal and offshore fisheries, can be inferred using the following: P(I) represents the prior probability of an industrial accident occurring in coastal and offshore fisheries; P(S) is the prior probability of an industrial accident occurring in stow net fisheries; P(I∩S) is the joint probability of both events occurring; P(S|I) is the posterior probability of an industrial accident occurring in stow net fisheries given that one has occurred in coastal and offshore fisheries; and P(I|S) is the likelihood function for an industrial accident occurring in coastal and offshore fisheries given that one has occurred in stow net fisheries.
This model represents conditional probabilities between variables structured as cause nodes and effect nodes using directed acyclic graphs to represent dependencies between variables with directed edges. One advantage of the model is that it enables predictions based on prior probabilities and backward inference. The use of Bayesian networks for modeling phenomena involving human and organizational factors has gained popularity in recent years (Trucco et al., 2008). These networks have been widely employed in constructing expert systems that account for uncertainty and have been extensively applied in maritime safety research (Hänninen & Kujala, 2014; Hänninen et al., 2013; Trucco et al., 2008).
Results and Discussion
Based on the incidence of industrial accidents among fishermen, stow net fishery stood out as a key area of concern. Table 1 summarizes the incidence among fishermen in coastal and offshore fisheries in South Korea between 2018 and 2022. Stow net fishery is undertaken in high-current areas, such as the Yellow Sea, using fixed nets with wide openings to catch fish swept in by the strong currents (NIFS, 2018). Over the five-year period, a total of 22,428 workers registered under the stow net fishery insurance program, out of which only 15 worked outside the Yellow Sea. This indicates that stow net operations are exclusively conducted in the Yellow Sea, unlike other fisheries that span multiple regions. Given the relatively high risk of industrial accidents in this area, stow net fishery was the focus of this study.
Under the Fisheries Act, South Korea categorizes fishery into licensed, permitted, and reported types. Permitted fisheries are further divided into coastal fisheries, which use non-motorized or powered vessels weighing under 10 tons, and offshore fisheries, which involve powered vessels weighing 10 tons or more. The stow net fishery is classified into coastal stow net fishery and offshore stow net fishery. Table 2 illustrates the status of industrial accidents among fishermen in these two categories over the study period. Analysis revealed that 32% of all accidents occurred in coastal stow net fishery, whereas 68% took place in offshore stow net fishery.
Coastal | Offshore | Total | |
---|---|---|---|
Number of accidents | 381 | 812 | 1,193 |
Ratio | 31.94% | 68.06% | 100% |
The insurance data include detailed classifications of accident types, which were identified based on the 41 categories defined by the Ministry of Employment and Labor. Common accidents in the stow net fishery included falls, slips, crush injuries, hit, struck-by injury, collapses, entrapment, and awkward postures. Table 3 represents the distribution of these accident types between coastal and offshore fisheries. The most common accident types in the stow net fisheries included slips, hit, and entangle. These three types of industrial accidents accounted for about 75% of all accidents in both coastal and offshore stow net fisheries.
The compensation insurance records include detailed information on risk influential factors, which are variables that impact the safety of fishing vessels (Wang et al., 2023). These factors include age of fishing vessel, gross tonnage, accident date and time, fishermen’s age and nationality, rank, work process, and environmental causes. To assess the relationship between industrial accidents and these risk factors in the stow net fisheries, the variables were categorized as shown in Table 4.
The association between the main types of industrial accidents and the identified risk factors in coastal and offshore stow net fishing were analyzed using the chi-square test (Table 5).
The results showed that 8 out of 9 safety risk factors, excluding the time of the accident, were significantly associated with the three main accident types. Fishermen’s age, nationality, work process, and environmental causes exhibited p-values < 0.001, indicating stronger associations compared to other factors. Further chi-square tests were conducted for the coastal and offshore stow net fisheries to examine the eight safety risk factors significantly associated with the three major types of industrial accidents (Table 6).
The three major types of industrial accidents were significantly associated with rank, work process, and environmental causes in coastal stow net fishery, whereas it was significantly associated with fishermen’s age, nationality, work process, and environmental causes in offshore stow net fishery. A Bayesian network was constructed to model these relationships (Fig. 2). The first node, or root node, represented the overall industrial accident rate of 4.49% for coastal and offshore fisheries of Korea over five years. The second node represented the rate of industrial accidents in stow net fisheries within the coastal and offshore fishery sector (9.38%) and was linked to the root node. In this structure, the root node acted as the parent node, whereas the second node served as the child node. Through this structure, the conditional probability of an industrial accident occurring specifically in the stow net fisheries, given that an accident has already occurred in the coastal and offshore fisheries sector, could be inferred. The third node differentiates between coastal and offshore stow net fisheries, with probabilities assigned for each. As the second node links to the third node, it transitions from being a child node of the root node to becoming a parent node of the third node. Based on this hierarchical structure extending from the root node to the third node, the posterior probabilities for industrial accidents occurring in either coastal stow net fishing or offshore stow net fishing could be inferred. This network connected to the final nodes representing the different types of industrial accidents, facilitating quantitative assessment of the risk associated with each major accident type.
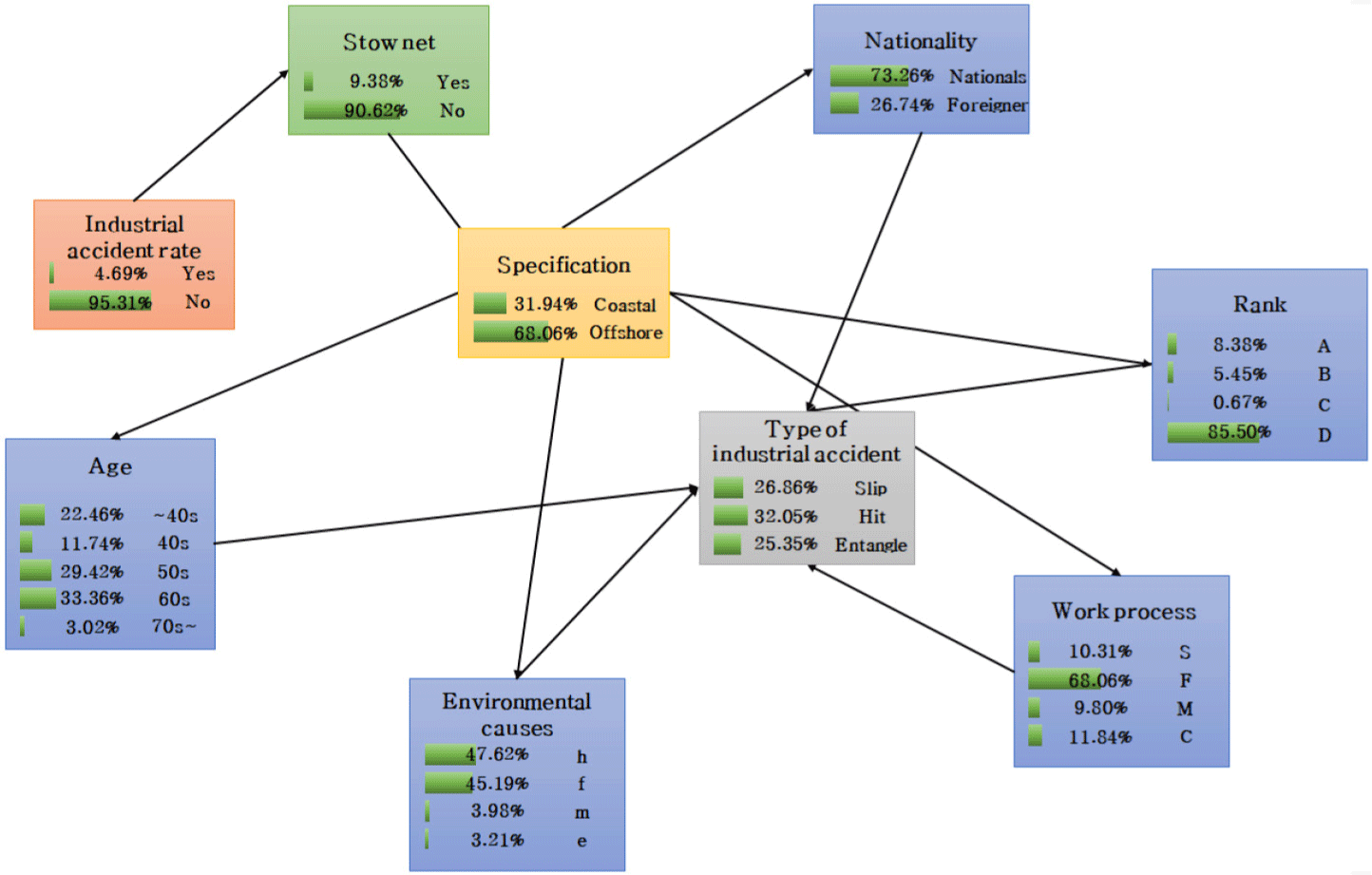
Using the Bayesian network, the quantitative risk for each accident type in coastal stow net fishery was calculated (Table 7). Officer-level roles, such as navigators and engineers, were excluded from the analysis, as there were no industrial accidents reported for these fishermen. The risk for seaman (D) was significantly higher, approximately 4.03 times that of Captain-equivalent (A). Based on 1,820 registered vessels and 381 insured crew members, the average number of crew members per vessel in coastal stow net fishing over five years was approximately 4.78. Since the crew primarily consisted of captains and general crew members, the risk associated with positions equivalent to head of department (category C) was notably low.
Rank | Slip | Hit | Entangle | Total |
---|---|---|---|---|
A | 0.00012 | 0.000082 | 0.000061 | 0.000263 |
C | 0.00000091 | 0.00000091 | 0.00000091 | 0.00000273 |
D | 0.00055 | 0.00026 | 0.00025 | 0.00106 |
Work processes | Slip | Hit | Entangle | Total |
---|---|---|---|---|
S | 0.00011 | 0.000028 | 0.000033 | 0.000171 |
F | 0.0004 | 0.00026 | 0.00026 | 0.00092 |
M | 0.000091 | 0.00004 | 0.000015 | 0.000146 |
C | 0.000063 | 0.000017 | 0.0000038 | 0.0000838 |
Environmental causes | Slip | Hit | Entangle | Total |
---|---|---|---|---|
h | 0.00048 | 0.000089 | 0.000026 | 0.000595 |
f | 0.00015 | 0.00024 | 0.00025 | 0.00064 |
m | 0.000015 | 0.000015 | 0.000015 | 0.000045 |
e | 0.000021 | 0.0000044 | 0.000025 | 0.0000504 |
In terms of work processes, fishing was associated with the highest risk across all accident types. The risk for accidents was 5.38, 6.30, and 10.98 times higher during fishing than that sailing, maintenance, and catch unloading, respectively, indicating that fishing is the most hazardous work process in terms of industrial accidents.
In terms of environmental causes, the highest risks were associated with slips on the vessel and hit or entangle by fishing gear. Hull-related accidents exhibited a cumulative risk that was over 10 times higher than those involving machinery or environmental causes. Quantitative risk assessments for offshore stow net fishing are shown in Table 8.
Nationality | Slip | Hit | Entangle | Total |
---|---|---|---|---|
Nationals | 0.00047 | 0.00037 | 0.00032 | 0.00116 |
Foreigners | 0.000026 | 0.000025 | 0.000025 | 0.000076 |
Work processes | Slip | Hit | Entangle | Total |
---|---|---|---|---|
S | 0.000077 | 0.00004 | 0.000035 | 0.000152 |
F | 0.00031 | 0.0003 | 0.00028 | 0.00089 |
M | 0.000083 | 0.000027 | 0.000021 | 0.000131 |
C | 0.000028 | 0.000032 | 0.000014 | 0.000074 |
Environmental causes | Slip | Hit | Entangle | Total |
---|---|---|---|---|
h | 0.00037 | 0.00013 | 0.0001 | 0.0006 |
f | 0.000098 | 0.00024 | 0.00022 | 0.000558 |
m | 0.0000091 | 0.000015 | 0.000022 | 0.0000461 |
e | 0.000021 | 0.0000052 | 0.0000047 | 0.0000309 |
Fishermen in their 50s and 60s faced the highest risk of accidents. The most common accident types in those aged 60–69 years were slips and hit, whereas it was entangle for those aged 50–59 years. In terms of nationality, national fishermen exhibited significantly higher risk, with a 15.26 times greater likelihood of accidents compared to foreign fishermen.
Fishing presented the highest risk across all accident types, with risks being 5.86, 6.79, and 12.03 times higher than those during sailing, maintenance, and catch unloading, respectively. Environmental risk patterns mirrored those in coastal stow net fishing, with hull-related slips and hit and entangle by fishing gear being most prevalent. However, the cumulative environmental risk was highest for hull-related incidents in offshore operations.
Conclusion
In this study, a quantitative risk assessment of industrial accidents was conducted using industrial accident data for fishermen engaged in coastal and offshore stow net fisheries over a five-year period in South Korea. Significant factors influencing accident occurrence were analyzed for coastal and offshore stow net fisheries using chi-square tests, and significant differences were observed between the two. In coastal stow net fishery, the risk to safety was significantly associated with rank, work process, and environmental causes, whereas it was significantly associated with fishermen`s age, nationality, work process, and environmental causes in offshore stow net fishery.
A Bayesian network was constructed using the identified factors to quantify the risk associated with the main types of industrial accidents. In terms of rank, the risk of accidents for seaman was 4.03 times higher than that for captain-equivalent and 388.28 times higher than for head of department, such as deck bosses or rigging chiefs, indicating a markedly higher safety risk faced by seaman. Fishing is associated with the highest safety risk across all types of industrial accidents. Slips were primarily associated with hull-related hazards, whereas hit and entangle were most frequently linked to fishing gear-related hazards. Among environmental causes, fishing gear posed the highest risk (0.00064), followed closely by the hull (0.000595), showing a risk 12–14 times greater compared to machinery and environmental causes.
In offshore stow net fishery, safety risk was highest among fishermen in their 50s and 60s, with a trend of increasing risk with advancing age up to the age of < 70. By industrial accident type, slips and hit were most common in those aged 60–69 years, whereas entangle was most common among those aged 50-59 years. The risk for national fishermen (0.00116) was approximately 15.26 times higher than for foreign fishermen (0.000076), highlighting a significant concentration of safety risk on national fishermen. Similar to coastal stow net fishery, fishing posed the highest risk across all accident types. Similar to the trend observed for coastal stow net fishery, slips were associated with the hull and hit or entangle were linked to fishing gear. However, in offshore stow net fishery, hull-related risk (0.0006) was 1.08 times higher than fishing gear-related risk (0.000558).
Stow net fishing involves deploying and retrieving heavy, high-resistance gear in areas with deep mud and strong currents. This results in both significant impact forces on the vessel and pronounced hull movement. The process of hauling in gear involves winding taut lines and nets with side drums and bow rollers against strong currents (NIFS, 2018). Additionally, muddy substrates from the seabed make the deck slippery during gear retrieval, increasing the risk of slips, struck-by injury, and entrapment. The findings of this study provide a risk assessment of industrial accidents among fishermen engaged in stow net fisheries in South Korea, offering important insights for safe operations in stow net fisheries, which involves high-risk factors.