Introduction
There is ample evidence indicating that humans have exploited aquatic resources for food since prehistoric times (Pitcher & Lam, 2015; Stepp, 2010). In many parts of the world, the role of fish in improving food and nutrition security has become increasingly recognized, leading to a significant increase in its consumption in recent years (FAO, 2022; Obiero et al., 2019a). Fish is rich in protein, lipids, and essential micronutrients such as vitamins A, D, and E, as well as minerals e.g., iodine and selenium (Balami et al., 2019). Fish and other aquatic animal foods are richer in omega 3 long-chain polyunsaturated fatty acids (n-3 LC PUFA), specifically docosahexaenoic (22:6n-3) and eicosapentaenoic (20:5n-3) acids, as compared to terrestrial animals (Tur et al., 2012). These n-3 LC PUFAs are important for human health including brain development and the prevention of cardiovascular diseases (Khalili & Sampels, 2018).
Integrating aquaculture output into food system policies and strategies has been promoted globally to transform the world’s agrifood systems, with several countries producing aquatic foods both for domestic consumption and export (FAO, 2022). Fish is one of the world’s most traded food commodities, accounting for about 10% of agricultural exports, with a global trade value that exceeds that of other animal products, and the major fish producers are developing countries, which account for more than half of global fish exports (FAO, 2022). Kenya’s aquaculture sector has grown to be one of the country’s most important economic sectors, supporting exports, rural development, and food and nutritional security (Obiero et al., 2019a). The rapid growth of the cage culture of Nile tilapia (Oreochromis niloticus) in Lake Victoria has resulted in inland freshwater aquaculture dominating fish production in the country, accounting for more than 80% of total fish production (Munguti et al., 2021).
Like any other food production sector, post-harvest losses plague the aquaculture sector. Fish are highly perishable and their quality begins to deteriorate immediately after they are harvested. Developing countries are more vulnerable to fish post-harvest losses due to inadequate storage and handling facilities, a lack of advanced processing techniques, insufficient processing facilities, a lack of technical capacity, and inadequate electricity and market infrastructure (Kaminski et al., 2020). These countries also have poor transportation infrastructure, which contributes significantly to the losses caused by fish transportation delays. Not only are fish post-harvest losses a major concern for public health and food security, but they also cause enormous financial losses for fish producers and traders (Keerthana et al., 2022).
The extent of fish post-harvest losses can be reduced by using various processing and preservation techniques. Fish preservation is paramount in reducing quality loss since it helps to maintain fish quality for longer periods (Sampels, 2015). There are numerous processing and preservation techniques available, ranging from simple traditional methods such as smoking, drying, and salting to more advanced modern and improved techniques such as damaging microbial DNA and pressure preservation (Sampels, 2015). Fish post-harvest losses can also be reduced through fish value addition, which has a variety of benefits such as opening up new marketing opportunities through product differentiation, increasing fish shelf life, maintaining fish quality, and improving the safety of fish products (Sampels, 2015). Fish preservation and processing of value-added fish products require modern and improved post-harvest technologies (Munguti et al., 2021). However, the commonly used preservation techniques in many developing countries such as Kenya are temperature-based methods and traditional methods like smoking and drying (Kyule et al., 2014; Munguti et al., 2021). Traditional methods, however, have limitations, and as a result, improved and modern technologies have been promoted as a solution to fish post-harvest losses (Keyombe et al., 2018).
To improve sustainable aquaculture production and marketing in Kenya, the government, in collaboration with various development partners and stakeholders, has increased its efforts to reduce fish post-harvest losses (Keyombe et al., 2018; Obiero et al., 2019b). Through these interventions, various post-harvest technologies, such as improved fish solar driers, improved fish smoking kilns, and improved fish processing and value-addition equipment, have been developed and promoted among fish traders across the country (Keyombe et al., 2018). However, user acceptance of these technologies has not been thoroughly studied. For instance, Obiero et al. (2019b), predicted the adoption of aquaculture technologies in Kenya, focusing primarily on production technologies, but did not take into account the post-harvest component. One of the areas of study prior to technology adoption is determining the degree to which new technology is accepted in society (Verma et al., 2018). Given the foregoing, it is vital to investigate the acceptance of improved post-harvest technologies among their intended users. As a result, the current study sought to assess improved fish post-harvest technologies (IFPT) acceptance among traders of farmed fish. Specifically, it sought to assess the socio-psychological determinants of fish traders’ intention to adopt IFPT. Therefore, the study will contribute to the body of knowledge by providing information on the social and psychological factors influencing the adoption of post-harvest technologies in the country and beyond. The study’s findings will be useful to policy makers and stakeholders in the fisheries and aquaculture sectors in reducing fish post-harvest losses.
The technology acceptance model (TAM), developed by Davis (1989), to explain the potential user’s behavioral intention (INT) to use technological innovation, was applied in this study. TAM has been suggested and used by various studies on the adoption of technology in aquaculture. Kumar et al. (2018), for example, highlight TAM as one of the best theories that can be used in understanding the dissemination of technologies in the aquaculture sector in their review of factors driving aquaculture technology adoption. In a study that sought to predict the adoption of aquaculture technologies among smallholder fish farmers in Kenya, Obiero et al. (2019b) described TAM as one of the models that consider more than a farmer’s characteristics or environment to have influence on their uptake of technologies but also recognizes the influence of psychological and motivational factors. Ulhaq et al. (2022) investigated the factors influencing shrimp farmers’ intention to use ICT using the combined TAM and theory of planned behavior model (Taylor & Todd, 1995). Ouko et al. (2022) also used TAM to investigate fish farmers’ intention to use black soldier fly larvae meal in Nile tilapia production.
TAM is widely used because it is simple, has high levels of prediction, and is regarded as a more flexible approach since it allows for the capture of some important psychological factors that influence an individual’s decision to adopt or reject a technology (Kumar et al., 2018; Silva et al., 2017). TAM is based on the theory of reasoned action (TRA), a psychological model proposed by Fishbein & Ajzen (1975). According to TRA, an individual’s INT is the driver of their actual behavior, which is determined by their attitude (ATT) toward the behavior and subjective norms surrounding its execution. This is to say that an individual’s ATT and perception of the behavior influence their behavior. TAM differs from TRA in two ways. First, two new constructs, perceived usefulness (PU) and perceived ease of use (PEOU), are introduced in TAM that are not found in TRA. Second, subjective norms are not included as determinants of INT in TAM.
TAM is based on the assumption that users are more likely to adopt a technology if they believe it will benefit them (Silva et al., 2017). As a result, TAM is founded on two constructs: PU and PEOU as determinants of an individual’s INT to use technology. PU is the degree to which a person believes that using a technology will improve their work performance, whereas PEOU is the degree to which a person believes that using a technology will be simple (Davis, 1989). According to Ajzen (1991), PEOU is one’s perception of how easy or difficult it will be to learn and use a technology. Furthermore, PEOU has a positive impact on PU (Davis, 1989). In other words, an individual who believes that using a technology requires no effort also believes that the technology is useful. As a result, a fish trader who believes that using IFPT is simple will be more likely to use them. On this basis, the following hypothesis was formulated:
H1: Perceived ease of using IFPT will have a positive and significant influence on their perceived usefulness.
Previous research has shown that in TAM, PU and PEOU determine one’s ATT towards the use of a technology (Ouko et al., 2022; Verma et al., 2018;). According to Fishbein & Ajzen (1975), ATT is defined as one’s positive or negative feelings toward a particular behavior. This implies that if a fish trader perceives IFPT to be easy to use and useful, they will be inclined to employ these technologies. The model also suggests that PU and PEOU have an indirect influence on INT via ATT, which is described as a mediator in TAM (Ouko et al., 2022). Previous research has found that PU and PEOU have an indirect effect on INT (Bagheri et al., 2021; Davis et al., 1989; Ouko et al., 2022). Based on this, the following hypotheses were formulated:
H2: Perceived ease of using IFPT will have a positive and significant influence on the ATT toward their use.
H3: Perceived usefulness of IFPT will have a positive and significant influence on the ATT toward their use.
H4: Perceived usefulness of IFPT will have a positive and significant indirect influence on the intention to use them.
H5: Perceived ease of using IFPT will have a positive and significant indirect influence on the intention to use them.
An individual’s ATT toward the use of technology influences their intention to use it, which is referred to as their INT (Davis, 1989; Fishbein & Ajzen, 1975). INT is defined as the extent to which a person has made cognitive plans to engage in or refrain from engaging in a particular behavior (Ajzen, 1991). Therefore, a fish trader who has a positive feeling toward using IFPT will have an intention to use them. Based on this, the following hypothesis was formulated:
According to TAM, other external variables such as age, gender, education, among others, can influence an individual’s intention to perform a given behavior (Davis et al., 1989). Previous research has shown that an individual’s socio-demographic characteristics have an impact on their INT (Kumar et al., 2018). Based on this, the following hypothesis was formulated:
H7: Socio-demographic characteristics of fish traders in Kenya will significantly influence their intention to use IFPT.
Fig. 1 shows the research model used in the current study, based on TAM and the formulated hypotheses.
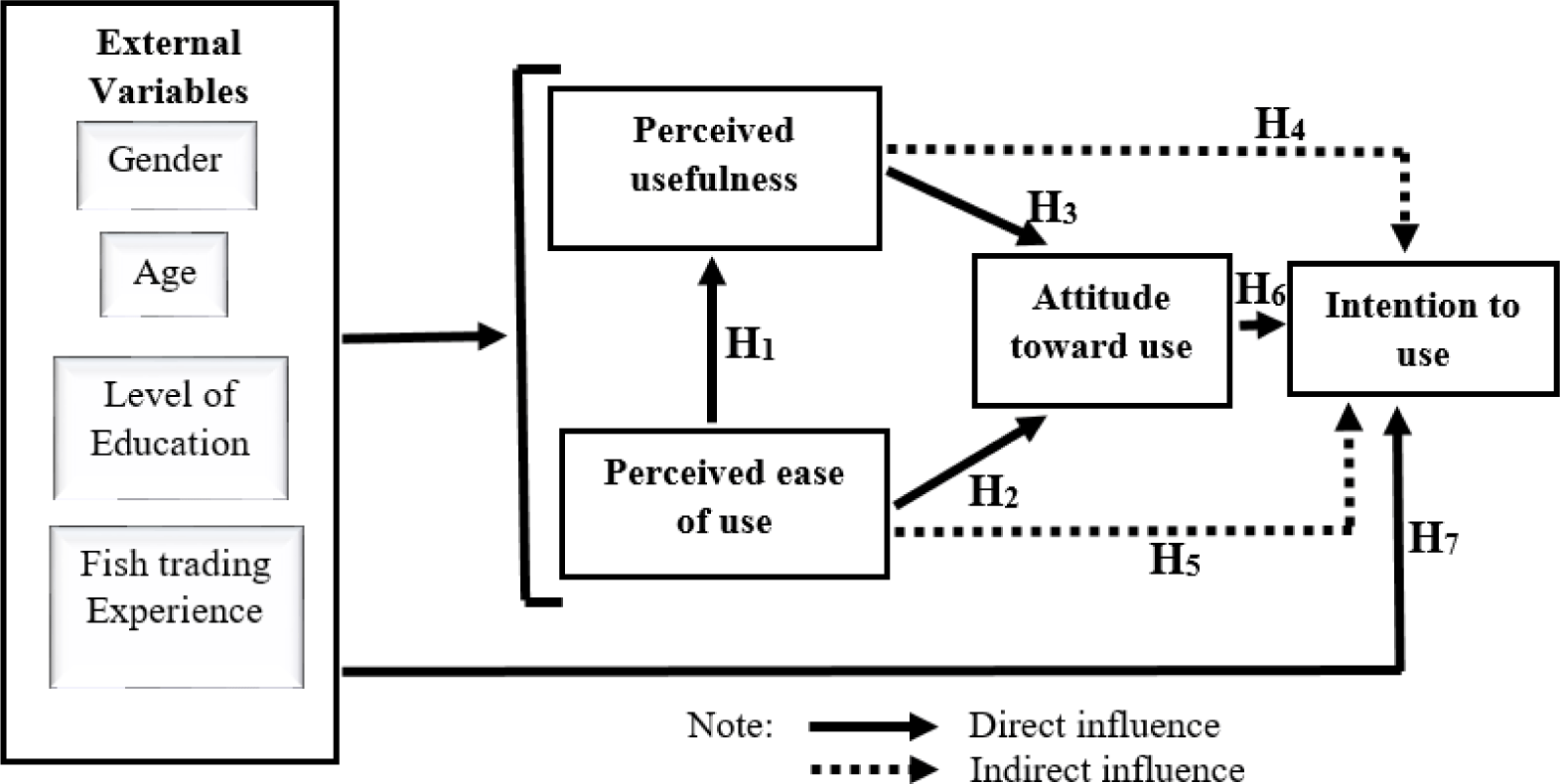
Meterials and Methods
The study was conducted in Kakamega, Siaya and Busia counties in Kenya. The study area was purposively chosen because of the high concentration of fish farming activities, particularly cage fish farming which is primarily practiced in Siaya and Busia counties, and pond fish farming in Kakamega county (Opiyo et al., 2018). Because of the high concentration of fish farming activities in the study area, there has been sporadic growth in the number of farmed fish traders, who are the primary target users of improved fish-post-harvest technologies in the country (Kawala et al., 2018).
A two-stage sampling method was used in this study. In the first stage, purposive sampling was used to select fish traders who were actively involved in trading of farmed fish. The second stage used systematic sampling to select traders from a list provided by fisheries officers in charge of fish marketing in the various counties.
A cross-sectional survey was conducted to collect first-hand information from the fish traders. A semi-structured questionnaire based on the TAM and inclusive of other socio-demographic components was developed to guide interviews. A week before the main survey, the questionnaire was pre-tested with ten randomly selected fish traders to avoid any probable usability issues, such as time management, complexity, applicability, and acceptability. The pre-test feedback was used to improve the questionnaire. The questionnaire was digitized using KoBoToolbox and the data collected with KoBoCollect mobile application. To reach the various respondents, trained enumerators were accompanied by various fisheries officers in the respective counties and sub-counties, who introduced them to the fish traders. The enumerators then conducted face to face interviews, each with a set number of respondents. Prior to the in-person interviews, informed consent was obtained from the respondents, indicating their willingness to participate in the study. The data collection tool was divided into two sections that captured: (1) the respondents’ socio-economic information and (2) their acceptance of IFPT. Participants indicated their level of agreement with the set of assertions about IFPT using a five-point Likert scale (ranging from 1 = strongly disagree, to 5 = strongly agree) based on the TAM constructs. The statements were created to reflect their perspectives and interests in adoption of IFPT.
All statistical analyses were done using IBM SPSS version 25 programme (IBM, Armonk, NY, USA). Descriptive statistics was used to analyze the socio-demographic characteristics of the respondents and the degree to which the respondents agreed or disagreed with the scale items used in the questionnaire.
Cronbach’s alpha reliability test was done to measure the reliability of the scale items used for each construct. Cronbach’s alpha measures how closely connected a group of items are to one another (Beavers et al., 2013). The acceptable values of Cronbach’s alpha are between 0.6 and 0.7 (Hair et al., 2010). To determine whether the dataset was suitable for a data reduction method, the Kaiser-Meyer-Olkin test (KMO) and Bartlett’s test of sphericity were used. KMO examines the relationship between two or more variables in a dataset. KMO values greater than 0.8 indicate adequate sampling, with 0.6 being the lowest acceptable value (Eyduran et al., 2010). The correlation matrix is compared to an identity matrix using Bartlett’s test of sphericity. It determines the variability of a data reduction method by examining whether there is a significant correlation between the correlation matrix and at least some of the variables in the dataset (Shrestha, 2021). This means that before performing data reduction, the Bartlett’s test of sphericity should be confirmed. If it is significant (p-value less than 0.01, 0.05 or 0.10), a data reduction method is appropriate (Eyduran et al., 2010; Shrestha, 2021). Principal component analysis (PCA) with varimax rotation was used for data reduction to select the number of components that would be retained in the measurement model. PCA is a data reduction technique that works best when the primary goal is to reduce the number of components because it assumes that all of the variance in the data is accounted for by the principal components (Budaev, 2010). PCA was used in the current study due to its better reliability and its capability to extract and characterize observations by multiple dependent variables that are correlated (Mishra et al., 2017). The Kaiser criterion of retaining eigenvalues greater that one was used (Eyduran et al., 2010; Mishra et al., 2017). Components with high loadings scores of at least 0.6 were retained to improve validity (Shrestha, 2021). The magnitude of variability of each variable retained in the two components from the PCA was shown by communalities. Communality is a metric that indicates how well the data reduction model accounts for the variance of a given item, with values ranging from zero to one, and values closer to one indicating better explanation of the variables by the components (Eaton et al., 2019). Communalities greater than 0.4 have been proposed as acceptable (Beavers et al., 2013; Mishra et al., 2017).
Linear regression analysis was used to test the hypotheses since it has been proposed as a reliable method of determining the relative influences of explanatory variables on the dependent variables in TAM (Davis et al., 1989; Eyduran et al., 2010). Four models were used in the regression analysis (Model 1, Model 2, Model 3 and Model 4). In Model 1, linear regression was performed to test hypothesis H1, where PEOU (independent variable) was regressed on PU (dependent variable). In Model 2, multiple linear regression was done to test hypotheses H2 and H3, where both PEOU and PU (independent variables) were regressed on ATT (dependent variable). In Model 3, using stepwise regression, PU, PEOU and ATT (independent variables) were regressed on INT (dependent variable) in testing hypotheses H4, H5 and H6 respectively. The stepwise regression was used to account for the indirect effect of PU and PEOU on INT through ATT. In Model 4, multiple regression was performed to test for hypothesis H7, where the socio-economic variables (gender, age, level of education and trading experience) were regressed on INT. A statistical significance at p < 0.05 was applied for all the relationships tested.
The items and statements presented in Table 1 were used as the indicators depicting the various TAM constructs used in this study.
Results
Table 2 summarizes the socio-demographic characteristics of the respondents in the study area. The vast majority of respondents were female (61%). They were mostly between the ages of 20 and 35 years, accounting for more than half of all respondents (51%), with the majority having only completed primary level of education (50%). The respondents’ years of trading experience varied, with most of them having between one and five years of experience in fish trading business (42.5%).
Table 3 presents the means and percentages of the traders’ responses on a scale of 1–5 (strongly disagree–strongly agree) to each of the items. In general, traders expressed a strong intention to use IFPT. The item with the highest mean (2.62) was INT2 (I would also use IFPT if other traders in my area used them) while the item with the lowest mean (1.97) was PU5 (I believe IFPT can bring more benefits to the environment than conventional methods). More than 60% of the respondents rated four or higher (agree and strongly agree) for all the items of perceived usefulness, perceived ease of use and ATT toward using IFPT. The intention to use IFPT was generally high, with more than 60% of the respondents giving a four or higher for two out of its three items. For INT2, 52.7% gave a three, indicating that the majority of the respondents were undecided whether they would also use IFPT only if other traders in their area used them.
Results of the Cronbach’s alpha reliability test for the variables that were gauged on a Likert scale are shown in Table 4. The highest Cronbach’s alpha coefficient was 0.817 for ATT while the lowest was 0.671 for INT. Since all of the coefficients were greater than 0.6, the Cronbach’s alpha signifies that the reliability of the scale items included in the questionnaire is satisfactory.
Variable | Cronbach’s alpha |
---|---|
Perceived usefulness | 0.693 |
Perceived ease of use | 0.748 |
Attitude | 0.817 |
Intention to use | 0.671 |
KMO was 0.914 and Bartlett’s test of sphericity was highly significant at (χ2 = 787.604, df = 91, p = 0.000). The KMO value which was higher than 0.8 shows that data collected was adequate for factor extraction and rotation. The significance of the Bartlett’s test of sphericity indicates that factor analysis was appropriate for this study.
As shown in Table 5, the PCA resulted in elimination of some items. Due to low loadings of less than 0.6, items PU1, PU4, ATT2 and ATT3 were eliminated. The analysis produced two components with eigenvalues of 6.007 and 1.164, respectively. Cronbach’s alpha coefficients were 0.846 and 0.653 for the components 1 and 2, respectively. The two principal components in the reduced datasets accounted for 51.28% of the total variance. The first component accounted for 42.91% of the variance while second component accounted for 8.31% of the variance. The Cronbach’s alpha coefficients for components 1 and 2 were satisfactory, indicating that the test for this sample of users’ intention to use IFPT has a high degree of reliability, according to the PCA. Additionally, every component examined in the analysis had a satisfactory loading of greater than 0.6. As demonstrated by the reduced dataset of two principle components, which explains 51.28% of the total variability, the PCA results effectively explain the data. All of the communalities were greater than 0.4, indicating that the items retained in the two components had an acceptable degree of variance. These findings point to a reliable measuring model.
The model summary of the regression analyses is as shown in Table 6. Model 1 had an R2 of 0.297, indicating that PEOU explained 29.7% of the variance in PU. Model 2 had an R2 of 0.503, indicating that 50.3% of the variance in ATT was explained by PEOU and PU. Model 3 had an R2 of 0.579, indicating that PU, PEOU and ATT explained 57.9% of the variance in INT. The R2 of Model 4 was 0.031, implying that the socio-economic variables only explained 3.1% of the variance in INT. This signifies that the socio-demographic variables are very weak predictors of INT.
Model | R | R 2 | Adjusted R2 | SE of the estimate |
---|---|---|---|---|
11) | 0.5452) | 0.297 | 0.292 | 0.51822 |
23) | 0.7094) | 0.503 | 0.496 | 0.47681 |
35) | 0.7136) | 0.579 | 0.573 | 0.42552 |
45) | 0.1777) | 0.031 | 0.004 | 0.60197 |
The hypotheses results are depicted in Table 7. The results show a positive and significant coefficient of PEOU on PU (β = 0.545; p = 0.000), supporting hypothesis H1. The coefficients of PEOU and PU on ATT were also positive and significant (β = 0.389; p = 0.000 and β = 0.418; p = 0.000, respectively), thereby supporting hypotheses H2 and H3. From the stepwise regression, the coefficient of PU on INT through ATT was positive and significant (β = 0.443; p = 0.000), thus supporting hypothesis H4. The coefficient of PEOU on INT through ATT was also positive and significant (β = 0.364; p = 0.000), thus supporting hypothesis H5. The stepwise regression yielded a positive and significant coefficient of ATT on INT (β = 0.615; p = 0.000), supporting hypothesis H6. None of the socio-demographic variables had a significant influence on INT at p < 0.05, thereby rejecting hypothesis H7.
Discussion
The objective of this study was to use TAM to understand the socio-psychological factors influencing fish traders’ intentions to use IFPT as a set of technologies. According to the descriptive statistics, majority of the respondents were females. This is due to the fact that females dominate the fish trading business in Kenya (Githukia et al., 2020). The findings revealed a considerably high proportion of youths (aged 35 and below) among the respondents. For many years, the marketing node of the aquaculture value chain has been dominated by people over 35 years (Farm Africa, 2019). However, due to efforts made by different stakeholders in pushing for the inclusion of women and youths in the value chain (Githukia et al., 2020), more youths have been actively involved in fish farming and trading business in the recent years, which could explain the current study’s findings. The low level of education among traders reported in this study is consistent with previous studies that have reported low education levels among aquaculture value chain actors, especially small-scale farmers, traders and processors (Munguti et al., 2021).
According to our findings, the statement “I would also use IFPT if other traders in my area used them” had the highest mean, with most of the respondents holding a neutral opinion on it. This suggests that the fish traders are unsure of the impact that other traders within their region may have on their adoption of the technologies. However, because this is a normative belief under subjective norms, it has no influence on the intention to adopt a behavior in the model (Davis, 1989). Furthermore, the findings show that majority of the respondents agreed with all other statements used in the study, indicating a generally positive intention to use IFPT.
The perceived ease of using IFPT has a significant influence on its perceived usefulness. Therefore, traders perceive these technologies as useful if they believe that using them is easy. These findings support previous research that found that a technology’s perceived ease of use influences its perceived usefulness (Ouko et al., 2022). Both perceived ease of use and perceived usefulness of IFPT have a significant influence on the ATT toward using them, implying that fish traders would have a positive ATT toward using the technologies if they believe they are useful and are easy to use. Bagheri et al. (2021) reported similar findings. According to the coefficients, perceived usefulness has a greater effect on ATT than perceived ease of use, thus supporting the findings of Flett et al. (2004) and Silva et al. (2017).
The current study’s findings also revealed that the perceived usefulness and ease of use of IFPT have significant influence on the intention to use them. The findings imply that fish traders would be willing to use the technologies if they perceived them to be useful and free of effort to use. They would not use a technology if it did not have a significant positive impact on their fish trading business. PU is thus important because it can be a barrier to IFPT adoption. Furthermore, PEOU should also be considered as an important barrier to IFPT adoption, as the likelihood of adopting a novel technology is low when users perceive the technology to be complex, despite its perceived usefulness (Bagheri et al., 2021). These findings are consistent with previous studies that found that perceived usefulness and perceived ease of use of technologies have significant influence on users’ intention to adopt them (Bagheri et al., 2021; Ouko et al., 2022). The findings are also consistent with Davis (1989) proposal that PU and PEOU are the primary factors determining the INT to adopt a technology.
The ATT toward use of IFPT has a significant influence on the intention to use them, indicating that the fish traders have better chances of using IFPT if they have a positive feeling toward using them. The findings also show that ATT is the most important factor influencing traders’ intentions to use the technologies, as indicated by the size of its coefficient. Previous research has found that users’ ATT is the most important determinant of their intention to adopt new technologies (Ouko et al., 2022; Verma et al., 2018). Therefore, the traders’ perspective on the use of IFPT is the most important factor underlying their intention to use them.
The current study found that fish traders’ socio-demographic characteristics are very weak predictors of their intention to use IFPT and have no influence on it. These findings suggest that a trader would not intend to use the technologies based on their gender, age, level of education or the number of years of experience in fish trading business. Several previous studies have found conflicting results regarding how these socio-demographic characteristics influence an individual’s acceptance of new technologies (Kumar et al., 2018; Obiero et al., 2019b; Ullah et al., 2022). However, the findings are consistent with Hasan et al. (2019) who found that an individual’s socio-demographic characteristics had a weak influence on their intention to use a technology. Additionally, Davis et al. (1989) reported that external variables have no significant influence on the intention to adopt a behavior and thus are poor predictors. Overall, our findings support Davis (1989) proposal that perceived usefulness and perceived ease of use are the primary determinants of technology adoption.
Conclusion
The present study improved our understanding of IFPT adoption by relying on an established model of technology adoption and focusing on personal perceptions as key factors in the adoption decision making process. It aimed to close a knowledge gap on the use of IFPT in the Kenyan context. The findings highlight some of the factors that influence fish traders’ intentions to adopt IFPT in Kenya’s most important regions of the farmed fish trading business, where traditional fish post-harvest technologies continue to predominate.
The model demonstrated that perceived usefulness is determined by perceived ease of use. ATT is determined by both perceived usefulness and perceived ease of use, which in turn determines intention to use. The traders’ intention to use the technologies was influenced by perceived usefulness, perceived ease of use, and ATT, with ATT being the most important determinant. This suggests that the use of these technologies in the fish trading business would increase significantly if traders had a positive ATT toward using them. Overall, the traders are enthusiastic about using IFPT. In the long run, the success of IFPT dissemination is largely determined by their cumulative acceptance. It can be concluded that traders will use IFPT when it is in their best economic interests.